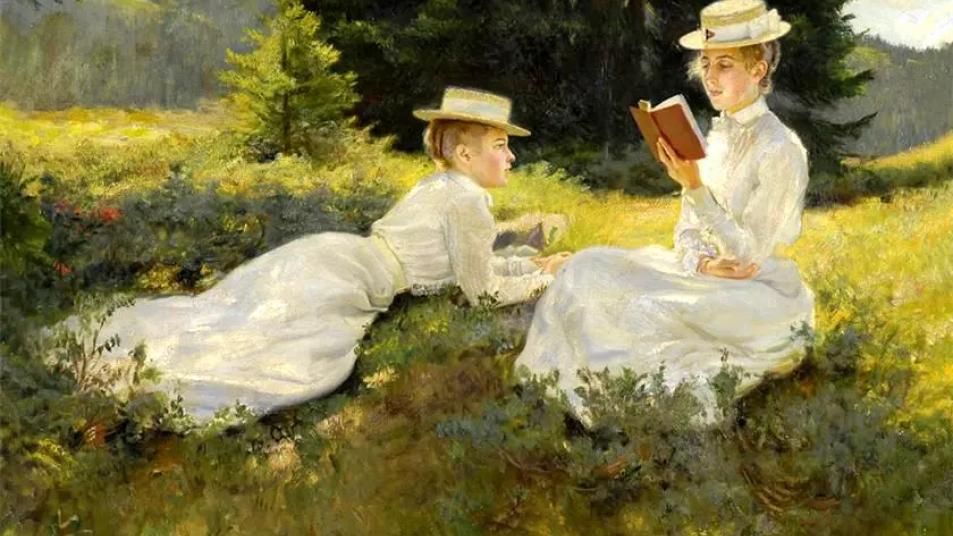
As part of the DHAI intensive week, Jean Barré led a project on the question of gender and more specifically on the representation of female characters in French literature. The participants in this project were Ismail El Hadrami, Ottilie Candau, Marc Noujaim, Milica Prugic and Pedro Cabrera Ramirez.
Let us first specify the approach of our work, which is part of the field of computational literary studies. It is based on the use of automatic language processing methods for text mining as well as machine learning methods to model concepts in large corpora of literary texts. One of the key concepts of the field is remote reading, theorized by Franco Moretti in the early 2000s. The goal is to explore the literary past with computational methods on massive corpora.
The idea is to include and take into consideration the thousands of texts that are today the forgotten ones of literary history.
This work was articulated around a general research question on the notion of genre. The objective was to evaluate the extent to which writers use gender stereotypes to describe fictional characters. This work builds on a 2018 study in the United States by Ted Underwood, David Bamman, and Sabrina Lee[1]
The global problem on the representation of the genre of characters necessarily induces to be able to locate the character, to recover its occurrences of appearance with if possible their textual context to finally assign a genre to the character. Different tools of Natural Language Processing exist to detect characters, and we will use the French version of an algorithm specifically developed for literary texts.
The main task of our work was to predict the gender of characters with the words that characterize them. To achieve this, we went through the different steps of computational literary studies, from corpus constitution, to metadata retrieval, through data annotation, information extraction and then statistical modeling of specific concepts, to results analysis. The time constraints of the week made us skip a few steps and this work is based on different pre-existing projects.
In particular, we use a corpus of 3000 novels from the 19th and 20th century, the Chapitres corpus. To identify
characters, we have implemented the Fr-BookNLP algorithm, developed in the Lattice lab. The latter detects named entities and solves co-referencing by aggregating different mentions of an entity under a single label.
We undertook gender annotation of our characters spotted by BookNLP. From a sample of 83 novels, we annotated the character gender for the ten main characters in each novel. To retrieve characterization information, we retrieved the ten surrounding words from each of our character mentions. These numbers are arbitrary and meet the time constraints of the intensive week. We had three labels, Male, Female, and Other. We assigned one of the three labels when we noticed gendered signs in the mentions of the characters, or in the surrounding textual content.
From the information present in the textual surroundings of each character mention, we undertook three different ways to extract information:
We use a support vector machine to supervisedly predict the gender of our characters. Let us specify that this study, for simplicity, is based on a binary conception of gender. This allows us a simpler computational approach as well as a shorter annotation phase in this limited time work week.
We implement the basics of machine learning, separating the data on which the model will train and the data on which we evaluate it. We thus measure the ability of the model to generalize on data it has never seen from the phenomena learned during training..
The results of the prediction are as follows: with the data in the form of bag of words or TF-IDF vectors, the model does not perform well. However, with Doc2Vec vectorization, our model performs at 85% efficiency, i.e., it recognizes the gender of characters in almost 9 out of 10 cases. These results seemed strong enough to continue our study and analyze phenomena on the whole corpus. We therefore undertook to predict the gender of the characters for our entire corpus.
The results of this projection are as follows: of the 27528 characters in the corpus, 17,604 (64%) are men and ,9924 (36%) are women. The fictional men are thus nearly twice as numerous as the fictional women. This result is already important and is significant about the over-representation of men in fiction. The factors that could explain this are numerous and difficult to fully identify. These figures are the witnesses of an invisibilization of women in the society even in the fiction, of a book market built by and for men in a patriarchal society.
To enrich our reasoning, we have posed a simple hypothesis that admits the gender of the male and female writers in our corpus as a factor that could explain these differences. The chapter corpus has in fact 417 authors, with 22% female and 78% male authors. Does having more male writers necessarily lead to an over-representation of male characters?
In Figure 1, we show the proportion of characterization by male and female authors. For female authors, there are 57% female characters and 43% male characters, while for male authors, 30 % of characters are female and 70 % are male. The gender of male and female authors is clearly a discriminating factor. While women have a fairly balanced representation of reality, authors are more likely to write stories with characters of the same gender as themselves.
When these results are projected over two hundred years of literature, the difference in treatment is all the more glaring. In Figure 2, the gap between male and female authors is enormous and of great stability over time. One does not note here effects of short-term fashions, but structuring lines of the literary and social history in the French-speaking context.
Finally, we wanted to plot specific words to assess their use in character descriptions. We calculated the set of occurrences of some words, if they occur around a mention related to a female character, we add one to the final value, otherwise we subtract one from this value. In this way we recover the gendered connotation of a given word. Figure 3 is a fairly simple example, with the word "monsieur" connoting male, the word "madame" connoting female, and the word "person" in a rather neutral space..
The next example is more interesting, with the words "office", "house", "room", which are respectively masculine, feminine and neutral. Gender stereotypes are visible here, with a different treatment of character space for men, more likely to work in an office and women to stay at home.
Here is a final example, with the words "god", "devil", "angel", which are masculine, feminine and neutral respectively
Rigorous analysis of these examples and their significance for literary history will be the subject of future work.
To wrap up, we were able, thanks to computational methods, to get a bigger picture of the representation of gender in the literary history. What we found is that the proportion of characterization of female characters depends strongly on the gender of the author. Male authors write half as much about female characters as female authors. We also tried to assess the extent to which literary characterization is related to gender stereotypes. While we did not have enough time to obtain insightful results, we did show that there are lexical contents closely related to gender role, and ultimately, to gender stereotypes.
[1] Ted Underwood, David Bamman, and Sabrina Lee, “The Transformation of Gender in English-Language Fiction”, Journal of Cultural Analytics, 3–2 (Feb. 13, 2018), doi: 10.22148/16.019.
Be the first to review this item, please login or register.
Sign-in Register