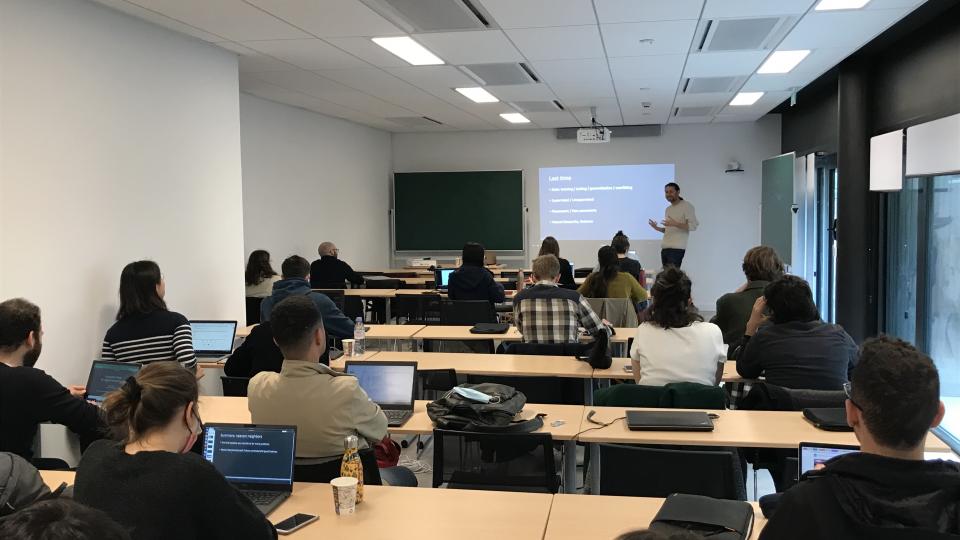
As part of the special “transverse program” of PSL, the DHAI group organizes with the help of PSL a special 1 week course on the topic of “Digital Humanities Meet Artificial Intelligence”. This course is open in priority to Master 2 students of PSL. It is also open to other students (Master and PhD) and researchers, subject to availability.
This week-long course will be split into three types of classes: theory, practice and project. During the project session, students will work in small groups toward a case study of practical importance. The final examination of the course will be a short presentation of the projects.
The practical sessions will feature:
Monday, March 28
Tuesday, March 29
Wednesday, March 30
Thursday, March 31
Friday, April 1
Course 1: Quantitative data analysis and cartography, Léa Saint-Raymond:
In 1944, the French state sequestered Kojiro Matsukata’s collection of paintings and sculptures as enemy property. The fate of this exceptional set was chaotic, until it was returned to Japan in 1959 - France having retained masterpieces such as Van Gogh’s bedroom and Gauguin’s landscapes. This course and related project aims to study these 360 artworks thanks to a computational analysis in order to eventually propose a visualization in digital humanities, through a collaborative website.
This course and related project offer training in computational data analysis. Students will receive the following theoretical and practical basics:
At the end of this training, students will be able to explore a corpus of data and analyze it from a quantitative and relational perspective. They will master the following software:
Course 2: Computer vision for the humanities, Mathieu Aubry:
Introduction to Computer Vision with a specific focus on Deep Learning. We will introduce the basic principles of Machine Learning and Neural Networks for Computer Vision applications. We will outline the specific difficulties of applications to historical and artistic data, standard use cases in digital humanity (image search, document segmentation, image recognition) and examples of specific projects on artwork price prediction, historical watermark recognition, pattern recognition and discovery in artwork datasets.
Course 3: Introduction to Natural Language Processing, Thierry Poibeau:
Natural language processing (NLP) is now everywhere and has attained a level of performance which makes it usable in practical contexts, especially for digital humanities projects. During this course, we will see a mix of theoretical and practical issues related to NLP. We will see why NLP is hard, and why recent approaches based on machine learning have made it possible to get efficient and robust modules, usable in digital humanities projects. Lastly, we will show practical implementations, making it possible to used advanced techniques at a reduced cost (from a computational point of view) for different tasks: part-of-speech tagging, named entity recognition and parsing, among others.
Course 4: DH/AI Tools and Astronomy, Matthieu Husson and Ségolène Albouy:
History of astronomy and AI: an overview: From Delambre (Histoire de l’astronomie ancienne, Paris,1817) through Neugebauer (History of Ancient Mathematical Astronomy, Berlin, 1975), the history of astronomy produced in Europe has a long relation to quantitative methods. Towards the end of the 1960s, this association intensified with the use of computers to assess ancient observations and computation methods (Poulle and Gingerich, 1968). Today, the rise of digital humanities and its coupling with AI opens new possibilities explored by different research projects. Based on a historiographical overview, this session will illustrate the current challenges emerging at the interface of history of astronomy, AI and Digital humanities with respect to artificial vision, text analysis and reenactment of ancient computations. We will discuss how AI can tackle ambitious challenges relating to issues as diverse as mathematical questions, genealogy of sources, transcription, diagram vectorization, and more.
A step aside: from document to data: Based on various examples from the previous session, we will explore how different datasets, exploitable by artificial intelligence algorithms, can be built to address, from historical sources, questions of various nature. We will evoke the stakes related to the transformation of a material document to its various digital representations: from the point of view of its acquisition, its modeling, its encoding, its “augmentation” or even its “simulation”. Finally, we will discuss what constitutes pertinent training datasets from a human sciences perspective as well as a machine learning perspective.
Project 1: Matsukata’s Confiscated Collection (Léa Saint-Raymond with Mathieu Rimet-Meille and Quentin Bernet)
In 1944, the French state sequestered Kojiro Matsukata’s collection of paintings and sculptures as enemy property. The fate of this exceptional set was chaotic, until it was returned to Japan in 1959 - France having retained masterpieces such as Van Gogh’s bedroom and Gauguin’s landscapes. This project aims to study these 360 artworks thanks to a computational analysis in order to eventually propose a visualization in digital humanities, through a collaborative website.
Project 2: Digital workflow from text retrieval to text alignment on a medieval French text (Lucence Ing)
This project aims to explore a digital workflow on a medieval French text, the Prose Lancelot. The workflow enables participants to discover deep-learning methods and automatic text alignment tools. It is made up of several steps : one step of text retrieval, from the pictures of the witnesses (versions of the same text), one step of structuration and enhancement of the text thanks to the use of a deep-learning linguistic tagger, and one step of alignment of witnesses (automatic collation). Then several explorations will enable us to understand the links between the different versions of the text.
Project 3: Exploring the Twittersphere of the French presidential election (Armin Pournaki)
We will learn how to design a sensible search query and collect Twitter data about the French 2022 presidential election. We will explore the data using interactive network visualizations which give a structural overview of the debate’s opinion clusters and their main actors. The textual content shared by these opinion groups will then be analyzed using natural language processing methods that you got to know in your theoretical course. This data exploration will allow us to generate hypotheses about the campaigning mechanisms of political actors on online social media.
Project 4: Analysing medieval astronomical diagrams (Tristan Dot & Ségolène Albouy)
This project will be focused on the automatic extraction and analysis of diagrams from astronomical manuscripts. After a first segmentation step (thanks to a convolutional neural network), we will use deep features in order to discover similar diagrams in our digitized corpus. We will explore how to cluster diagrams according to content and shape, without considering “style,” in order to produce the first AI-assisted critical edition of medieval astronomical diagrams.
Be the first to review this item, please login or register.
Sign-in Register